Supporting Graduate Students' Academic and Professional Success
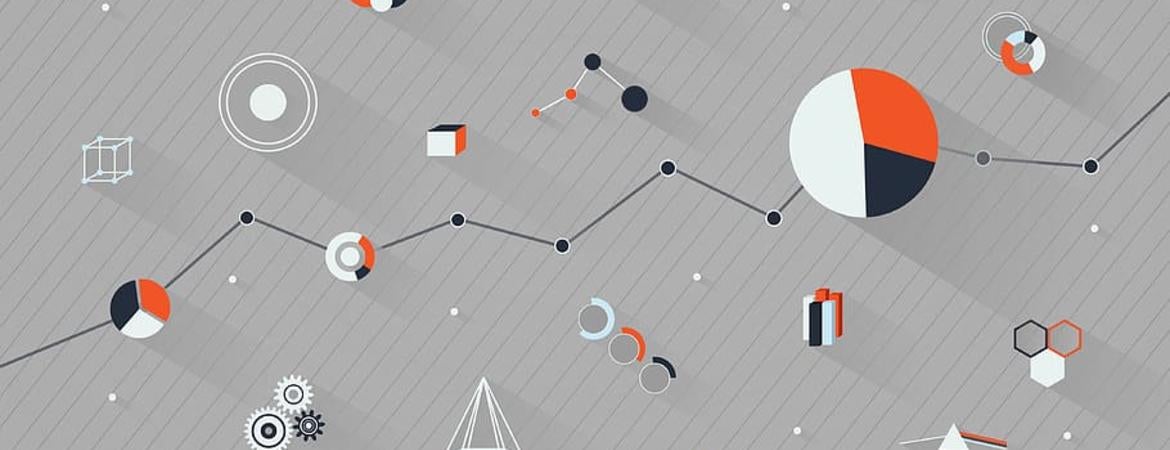
Friends,
It was nearly 100 years ago that R.A. Fisher, a founder of contemporary statistical thought, published Statistical Methods for Research Workers (Fisher, 1925). This popular and deeply influential book explains data science in a way that is accessible to practitioners, both in the early 20th century and in the modern day. At the time of the book's release, the study of statistics was relatively young. Many of the techniques in Statistical Methods are still used widely today, including goodness-of-fit tests, correlation measurement, analysis of variance, and more. In the last century, statistical science has grown nearly as fast as the data on which it operates. Data-driven research is accepted by many as the best path to an accurate and objective understanding of the world. Indeed, data are the observable facts that the world has given to us so that we can better understand it. Interestingly, the word data derives from the Latin verb dare—to give. Today, many of us—if not most—are required to perform quantitative analysis in some form. Statistical methods have infiltrated nearly every corner of scientific research, and it is important that we know how to use them. Thankfully, the curators of data science have continued to emphasize the application of their craft. Books like Fisher's have become abundant, and the internet has caused a profusion of resources for modern practitioners. In the discussion that follows, we will review some of these resources to help graduate students both during and following their studies at UCR.
[Image description: The words “Pave the Way for a Data-Driven Future” appear against a dark green background.]
Pictured: Pave the Way
During Graduate School:
Most research universities have resources for data computation and analysis. At UCR, we have several resources for those in need of assistance in these areas. First, and perhaps most obviously, we offer classes in statistics and data science. For many graduate students, it is compulsory to take advantage of this resource. Program requirements, particularly in STEM fields, often incorporate courses in statistics, computer programming, or data science. I encourage graduate students of all disciplines to take a course in statistics—especially if they will ever perform research in their field. I have encountered many graduate students from our campus community in my introductory statistics courses. These courses are great for students with a bit of mathematical background who have never done quantitative analysis. We also offer courses tailored to specific scientific disciplines. For instance, the Department of Statistics is offering the following courses in Spring 2022: Statistics for Business, Probability and Statistics for Science and Engineering, and Elements of Data Science. For students with a bit more background in programming, the Computer Science department offers courses in machine learning. UCR also offers support outside the classroom. Our Graduate Quantitative Methods Center (GradQuant) offers free training in quantitative methods for UCR graduate students and postdocs. GradQuant provides individualized consultations and regular workshop programming on a variety of topics. We also offer training in digital research methods for programs in humanities and social sciences. The UCR Library is another great resource. The library carries many books on statistical methods. The library also has on-site staff who are available to help with data-related questions. Kat Koziar, our data librarian, holds virtual office hours each week which students can attend without an appointment to ask questions about data cleaning, data visualization, data analysis, coding, and more. GradQuant offers a similar service. For formal research collaboration with faculty, UCR has the Statistical Consulting Collaboratory. This resource specializes in statistical consulting and modeling in a way that is academically rigorous. The Collaboratory offers 10 hours of free consulting services for all UCR faculty, staff and students. After 10 hours, on-campus clients can schedule additional time at a rate of $62 per hour.
[Image description: Milk pours from a carton into a bowl positioned against a cereal box picturing a robot. Number-shaped cereal floats in the bowl atop the milk. Above the image are the words: “I eat data for breakfast”]
Pictured: Ultimate Breakfast
After Graduate School:
Utilizing resources for data science outside of academia can be confusing. The issue is not a lack of resources, but an excess of resources with tremendous variance in their utility. Weeding out resources that are unhelpful—or simply erroneous—can be difficult for those being introduced to quantitative analysis. For those who are completely new to statistics, there are several free resources with which to get started. Khan Academy offers courses in Statistics and Probability, which are at the high school/college level. They also have a course in Computer Science that contains a module on data analysis. These resources are delivered in a way that is approachable and aesthetically pleasing; they are great for self-teaching. To gain a more in-depth understanding, I recommend reading books. Online forums are valuable for quick questions and for building general intuition. I have reviewed many of the Wikipedia pages on statistical methodology, and most are accurate and readable. Wikipedia actually has an entire collection of articles on machine learning and data mining (insert usual disclaimer about using Wikipedia for research). However, I find that the best way to learn a new topic is by reading published works that have been thoroughly reviewed and vetted by peers. For beginners to data science, OpenIntro Statistics is a good option. OpenIntro is a nonprofit organization staffed by a team of fellows and volunteers with a mission to make educational products that are “free, transparent, and lower barriers to education.” The organization offers several books on statistics, which are all free to download with an optional donation. OpenIntro Statistics 4th Edition covers everything from “what are data” to advanced regression and analysis of variance. I have used this resource as a textbook for teaching statistics courses at UCR. At over 400 pages in length, it provides a comprehensive overview of many important statistical topics, along with a plethora of useful exercises. Those who want more in-depth and formal training may be interested in university extension programs. Many university extensions offer fully-online programs in data analytics or related subjects. Some of these programs are completely free, some are only free if students waive their right to a certificate—i.e., if they audit the program—and some are quite expensive. Some require an application, and some do not. The UCR Extension offers courses in data analytics and applied statistics. All the aforementioned resources require some effort to reap their benefits. However, they all have the potential to help postgraduates develop their quantitative skills and realize their professional goals.
[Image description: A plot titled “How I feel about Mountains”, plots “time” against “awe”. The plot is traced out to form the silhouette of a mountain.]
Pictured: How I Feel about Mountains
In the preface to Statistical Methods for Research Workers, R.A. Fisher laments that traditional statistical machinery, with its elaborate large-sample theory, is “wholly unsuited to the needs of practical research. Not only does it take a cannon to shoot a sparrow, but it misses the sparrow!” His book was one of the first to promulgate statistical theory within the scientific community in an approachable way. These days, practitioners have a virtually endless collection of resources to draw on for their quantitative analysis. We have reviewed just a few of these resources. I hope some will be helpful to you at UCR and Beyond.
References:
Fisher, R. A. (1925). Statistical Methods for Research Workers. Oliver and Boyd.